Exploring Machine Learning Basics: Models and Trends Reshaping the Industry
Understanding Machine Learning Models: An Introduction
What Are Machine Learning Models?
Machine learning models are algorithms that allow computers to learn from data. These models find patterns in data without being directly programmed. They improve with experience. Machine learning models include supervised, unsupervised, and reinforcement learning. They are key in AI, helping to make smart decisions without human help. These models drive advances in many fields, from healthcare to finance.
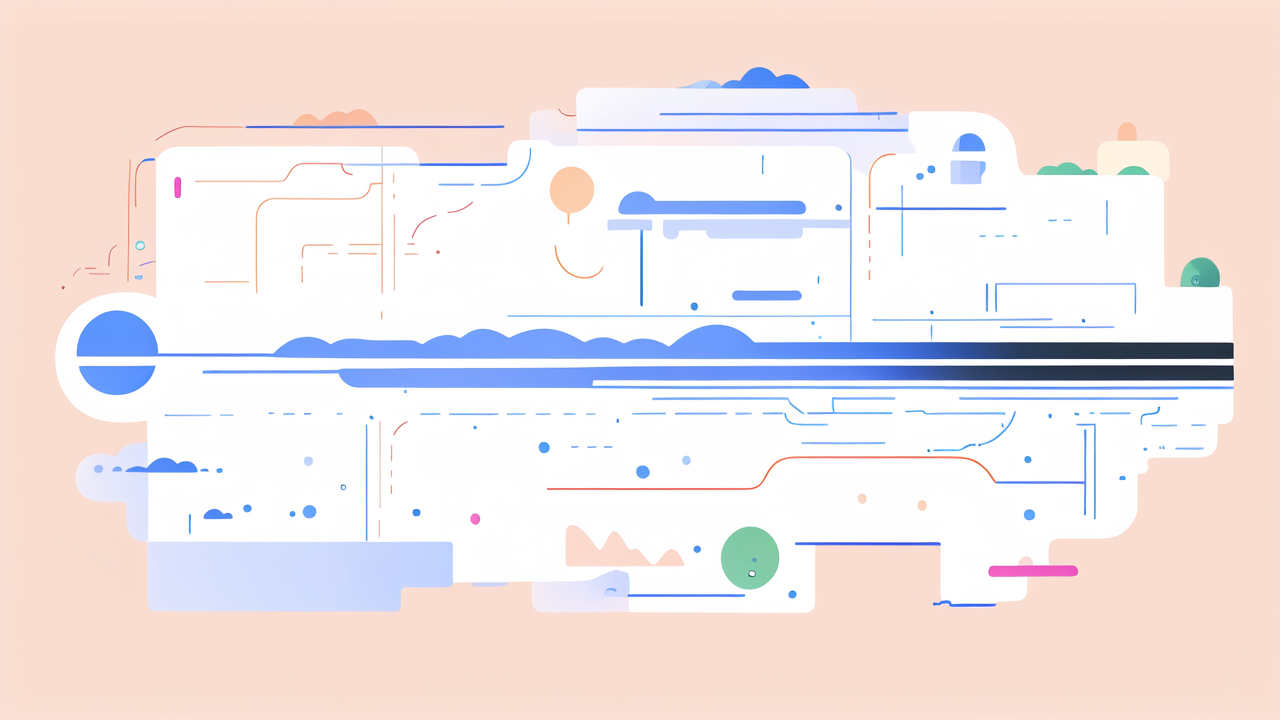
The Role of Machine Learning Models in Data Analysis
Machine learning models dig deep into data. They spot patterns we might miss. These patterns can help make smart decisions. In business, models predict trends and customer habits. In science, they make sense of complex data. They guide us in making choices based on solid data analysis. This improves accuracy and efficiency.
Key Types of Machine Learning Models
In machine learning, models are the systems that learn from data to make decisions. There are several key types of models, each with unique methods and uses. Below is a list of the major types:
- Supervised Learning Models: These models learn from labeled data. They predict outcomes based on past data.
- Unsupervised Learning Models: They find patterns in data without labels. These models identify groups and commonalities.
- Semi-Supervised Learning Models: A mix of the above two. They learn from both labeled and unlabeled data.
- Reinforcement Learning Models: These models learn by trial and error. They make decisions and learn from the results of those actions.
- Deep Learning Models: A subset of machine learning. These models use neural networks to analyze complex data patterns.
Each type of model plays a crucial role in solving different problems. They turn data into insights and action.
The Evolution of Machine Learning Models
The History of Machine Learning
The history of machine learning dates back to the mid-20th century. Early concepts of computers learning from data emerged around the 1950s. These ideas took shape with the development of neural networks and algorithms. By the 1980s and 1990s, machine learning evolved with the improvement of computational power. Progress in storage and processing allowed for complex data analysis. Aiding this evolution were breakthroughs in theoretical understanding. Machine learning’s history is marked by steady advancements that set the stage for today's AI. This continuous growth reflects a deepening grasp of its potential impact.
Recent Advancements in Machine Learning Models
In recent years, machine learning (ML) has seen significant advancements. These include the rise of deep learning, which mimics the human brain's neural networks. Advancements include reinforcement learning, where models learn from interactions. Transfer learning is another trend. It lets models apply knowledge from one task to another. The use of large datasets, or 'big data', has also improved ML models. The integration of ML with the Internet of Things (IoT) is another key trend. Together, they create smart, connected systems. Lastly, autoML is emerging. It automates parts of the ML process, making it more accessible.
How Machine Learning Models Are Shaping the Future of AI
Machine learning models are not just a part of AI; they are driving its future. They enable systems to learn and predict without being explicitly programmed. As AI evolves, these models become more advanced, improving their ability to handle complex tasks. From self-driving cars to personalized medicine, machine learning models are at the heart of AI innovation. They are embedded in various systems, enhancing their intelligence. The growth of big data and computational power fuels these advancements. We are moving towards AI that can reason and make decisions like humans. This progress will change how we live and work. Machine learning models are key to this transformative future.
Applications of Machine Learning Models Across Industries
Machine Learning Models in Healthcare: Enhancing Patient Care
Machine learning is changing healthcare. It helps in many ways. AI can spot diseases early. It makes sense of big data fast. Doctors use it to choose better treatments. Patients get care tailored just for them. Hospitals use AI to cut costs and save time. ML models predict patient risks. They also help manage health records safely. This tech is vital for modern medicine. It improves health outcomes. It also offers care that was not possible before.
The Impact of Machine Learning Models on Retail and E-commerce
- Personalization: E-commerce uses machine learning to offer custom product suggestions.
- Inventory Management: ML models forecast demand to optimize stock levels.
- Customer Service: AI chatbots powered by ML enhance user support.
- Fraud Detection: Retailers use ML to spot and prevent fraudulent transactions.
- Price Optimization: Dynamic pricing algorithms adjust prices based on supply and demand.
- Marketing Insights: Machine learning analyzes customer data to improve marketing tactics.
- Supply Chain Management: ML enhances logistics through predictive analytics.
Machine Learning Models in Telecommunications: Network Optimization
Machine learning is reshaping telecoms. It optimizes networks for better service. Smart algorithms predict and fix network issues. This boosts speed and reduces outages. Telecoms use machine learning for traffic forecasting. This prepares networks for high demand times. It also helps in fraud detection, saving companies losses. AI analyzes call data, spotting unusual patterns. It prevents fraud before it happens. Machine learning also personalizes customer experiences. It suggests plans based on user data. This makes customers happy and loyal. In summary, AI makes telecoms smarter, faster, and safer.