The Evolution of ML Classification Algorithms in the Tech Industry
The Rise of ML Classification Algorithms in AI and Automation
Understanding ML Classification
Machine Learning (ML) classification is a core function in AI. It sorts data into groups. This makes machines 'smart'. They can tell cats from dogs, spam from genuine emails, or if a bank transaction is fraud. To do this, ML uses training data to learn patterns. Then, it applies what it learns to new, unseen data. As it gets more data, it gets better at classifying. This is key to automation. It helps businesses speed up tasks and cut errors.
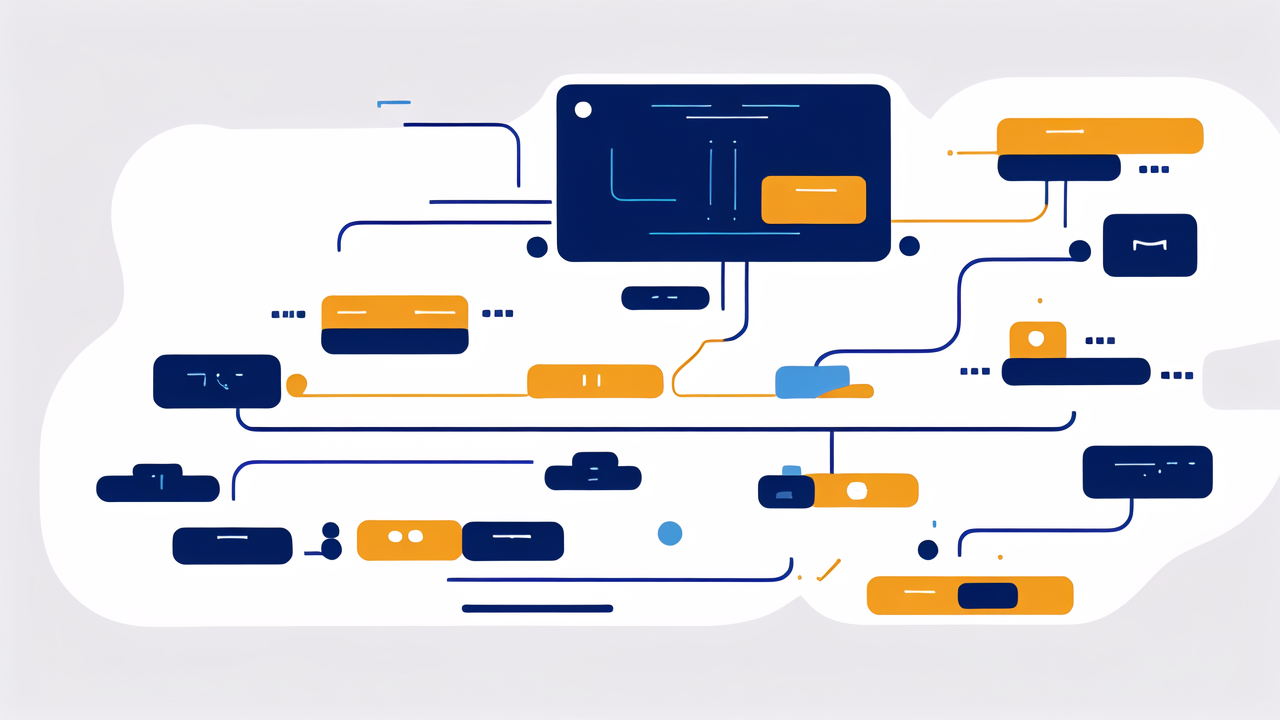
The Role of AI in Modern Business Operations
Artificial Intelligence (AI) has become a core part of modern business. It makes operations faster and smarter. AI helps in sifting through data to find patterns. This skill is vital in many areas like marketing and fraud detection. With ML classification, AI systems can now learn and improve over time. This means they make fewer mistakes and save companies lots of money.
Companies now rely on AI to understand their customers better. This helps them create new products and services that people love. ML classification also makes it easier to manage large volumes of data. This is important in industries like healthcare and finance. As a result, businesses can offer better services and make more informed decisions.
The Impact of Accurate Classification on Automation
The ability to classify data accurately is a pillar of successful automation. It enables machines to make smart decisions without human help. When classification is correct, automation becomes more reliable, efficient, and cost-effective. ML algorithms can spot patterns in vast amounts of data. This helps in refining processes across industries. For example, in manufacturing, it can predict machine failure. In healthcare, it can diagnose diseases faster. Accurate ML classification is key to advancing automation in the tech industry.
Comparing Traditional Classification Methods with Modern ML Algorithms
The Shift from Rule-Based to Data-Driven Classification
Traditional classification relied on preset rules. Humans had to write these rules based on their knowledge. But this method had limits. It couldn't handle complex or changing data well. Modern ML algorithms offer a new way. They learn from data instead of following set rules. This means they can adapt and improve over time. They find patterns and make predictions based on those patterns. Data-driven approaches are more flexible. They can manage large and dynamic data sets. Businesses now prefer ML methods for these reasons. They provide better results in less time.
How ML Algorithms Are Changing the Game in Various Industries
Machine Learning (ML) algorithms have drastically altered the landscape of various industries. They facilitate more advanced, scalable, and personalized solutions compared to traditional classification methods. Here are a few ways ML is influencing different sectors:
- Healthcare: ML algorithms help in diagnosing diseases with higher accuracy and speed.
- Finance: They are used in fraud detection, risk assessment, and customer segmentation.
- Retail: ML enhances customer experiences through personalized recommendations.
- Manufacturing: Predictive maintenance and quality control are made efficient with ML classification.
- Transportation: In improving safety, ML algorithms play a role in vehicle classification and traffic management.
These examples show how ML algorithms are not just changing, but revolutionizing industry practices.
The Benefits of Implementing ML Classification in Businesses
- Improved Decision-Making: ML algorithms analyze vast data. This leads to smarter business choices.
- Enhanced Efficiency: Automation with ML reduces human error. It also speeds up processes.
- Better Customer Experience: Personalized services are possible with ML. They increase customer satisfaction.
- Cost Reduction: ML algorithms lower operational costs. They do this by optimizing resources use.
- Competitive Advantage: Companies using ML can stay ahead. They can quickly adapt to market changes.
- Scalability: Businesses can scale services easily with ML. This helps manage growing data loads.
Industry Trends and Future Developments in ML Classification
Innovations Shaping the Future of ML Classification
As we advance further into the tech era, ML classification algorithms are undergoing rapid evolution. Key innovations influencing this growth include advancements in deep learning, such as convolutional neural networks (CNNs), which have proven effective in image and video classification. Additionally, reinforcement learning is being increasingly leveraged to enhance the decision-making capabilities of AI systems. The growth of big data has also fueled the development of scalable algorithms capable of handling vast amounts of information. Furthermore, the integration of transfer learning enables models to apply knowledge from one domain to another, significantly reducing training time and resource consumption. Another trend is the use of self-learning algorithms that iteratively improve with minimal human intervention. These innovations are not only shaping the present but also paving the path for future breakthroughs in ML classification.
The Importance of Continued Research in ML Algorithms
Continuous research in ML algorithms is crucial for progress. This work keeps AI advancing. It leads to more robust and versatile solutions. A key aspect is developing algorithms that can adapt to new challenges. This means they learn from more diverse data. It ensures that classification tools remain effective and efficient. Over time, such research may lead to groundbreaking discoveries in AI and beyond. The goal is smarter AI that can understand and interact like never before. This research is the backbone of AI's future growth.
Predicting the Next Big Thing in ML Classification Technology
Predicting the future in tech is often a challenge. Yet, in ML classification, some trends stand out. We're seeing a push towards integrating AI with neuroscience. The aim is to create 'deep learning' models that mimic how humans learn. Another big area is the use of quantum computing. It could vastly speed up data processing in ML. Then there's the concept of 'Explainable AI' (XAI). This is all about making AI's decisions clear to users. Lastly, 'AutoML' is on the rise. It's where AI itself helps design better ML models. Each of these could be the next big leap in ML classification.